Transform customer data into predictive insights with an AI CDP
You don’t need an army of data scientists to generate predictive models from your customer data, or complicated pipelines to translate this intelligence into business value. mParticle is democratizing AI adoption, and turning what was once a pipe dream into a catalyst for your pipeline.
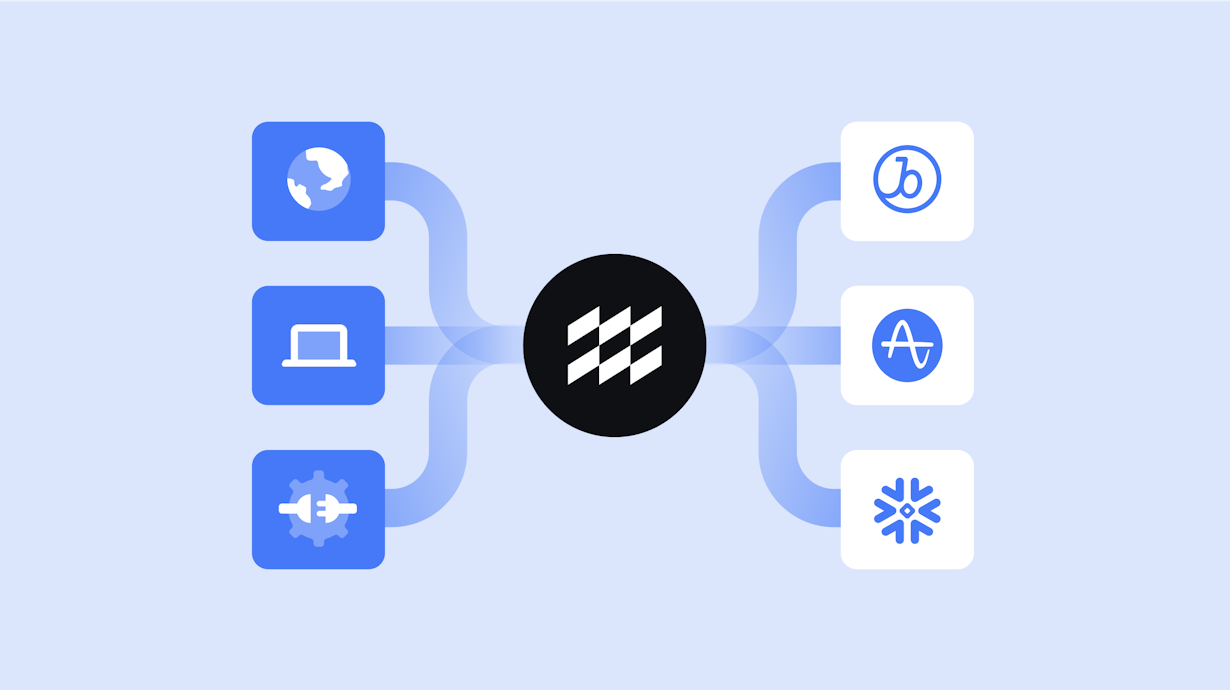
These days, you probably hear the phrase Artificial Intelligence uttered quite a bit. It’s the wave of the future, the next phase of digital transformation, and a train you don’t want to miss. Every day, it seems like a new software company emerges with an AI-based offering, or a new white paper on the subject surfaces in your LinkedIn feed. You know it’s big, you know it’s transformative. What you might not know, though, is how you can actually use AI to build better relationships with your customers, improve your product experiences, and grow your business.
What exactly is artificial intelligence?
Artificial intelligence (AI) is the subset of computer science that deals with giving computer programs the ability to make decisions and reason about things in a way similar to humans. In most cases, computer programs receive an input, run it through a set of human-generated instructions, and produce an output. AI programs can take that same input, make decisions or inferences about it based on other information, and provide an “intelligent” reaction or output as a result of this reasoning process.
A brief background on artificial intelligence
Before we get into how AI can transform the way you leverage customer data, let’s briefly (I promise) discuss where this concept came from. In his 1950 paper “Computing Machinery and Intelligence,” Alan Turing asked his readers to consider a question: Can machines think? Throughout this work, which many consider to be the conceptual birth of AI, Turing lays out a thought experiment called “The Imitation Game” that imagines a human test subject attempting to distinguish between a machine and a person in terms of their reasoning and thinking capability. In laying out this scenario, Turing argues that there is no reason why machines could not one day have the ability to “think” the way humans do.
Since Turing planted this seed over 70 years ago, computer scientists have been working to put his theory into practice in the form of real programs capable of intelligent reasoning. Today, AI is shaping our lives in real, tangible ways––automated manufacturing robots, Natural Language Processing tools, smart assistants, self-driving cars, and business intelligence are just a few examples of artificial intelligence at work in the world today.
How can AI enhance the way companies leverage customer data?
Collecting high-quality first-party data from your users across all of your digital touchpoints and tying this data into a unified view of your customers is the foundation of any successful growth, engagement, or optimization strategy. But today’s consumers have such high expectations for personalization that in order to stand out among competing brands, it is no longer enough to base personalization decisions on historical data alone. Customer-centric teams need to engage with customers not based on what users have done in the past, but what they are likely to do in the future.
This is where AI steps in to transform a marketing team’s entire customer engagement strategy, or a product org’s whole approach to in-app personalization. AI adds a layer of intelligence and insight on top of what your data can explicitly tell you about your customers, and gives you the ability to execute use cases with your data that would have been impossible using static information.
Some examples of AI-powered data activation use cases include:
- Prescriptive churn reduction: Instead of sending a re-engagement email trying to win back a customer who has churned, you can predict those who are likely to churn and intervene before they do so.
- Conversion predictions: Rather than showing targeted ads to all of your prospects, you can focus your ad spend on those with a high likelihood of converting.
- In-app recommendations: Using predictive modeling, you can show your customers products and offers that have a high likelihood of resulting in purchases.
- Dynamic decisioning: Don’t waste discounts and coupons on customers who already have a high likelihood of converting––use propensity scoring to save offers for the customers they will impact the most.
What prevents growth teams from adopting AI?
Given the advantages that AI-powered personalization has over more traditional usages of customer data, one might wonder why every brand in the world is not currently leveraging AI insights in every campaign they run and decision they make. There are many reasons for this, and we’ll address a few of those here.
Lack of data science resources
Data scientists––that is, engineers with the skills and experience to generate predictive insights from datasets––are highly sought after and hard to come by. Maintaining a team of these folks on staff may not be feasible for many small- to mid-sized companies. Even at larger organizations that do have these dedicated resources, the turnaround time for these teams to deliver on requests for predictive insights is often too long for marketing and product teams to leverage in real world use cases.
Say, for example, a marketing team wants to send a specific trial offer to a subset of users who are likely to convert. When these marketers ask their data science team to run the predictive analytics and create an audience of likely-to-convert prospects, the data scientists will do so using a dataset that reflects prospects’ current state at the time of this request. By the time they generate the audience, some of these customers may have converted already, meaning these customers will receive an irrelevant offer from the company.
AI insights stay locked in a single platform
Many data activation vendors on the market, like email marketing tools, product analytics solutions, and customer engagement platforms, offer some form of AI insight-generating capability. While this may help marketing teams engage more intelligently with their customers on that platform, there are drawbacks to adopting AI downstream in the data stack. First, the insights this tool generates will be limited to the data that this one system receives, which will likely not reflect a complete view of the customer. Also, as far as AI insights go, what happens in downstream tools stays in downstream tools––in other words, these predictions won’t have any utility in other tools for data activation, orchestration, or analysis.
Overly technical AI solutions don’t teach growth teams to fish
As is the case with any other customer data set, it is growth, marketing, and product teams that are the end users of AI-powered insights. This being the case, the AI solution that a brand adopts should not have too high a technical bar as to preclude go-to-market teams from being able to easily and flexibly leverage it. What’s more, if AI-powered insights are simply delivered as a black box, and these end users have no visibility into how the models are generated, their utility will be very limited. To borrow from the “teach a man to fish” adage, if you give a marketing team a churn risk prediction, they will prevent losing customers for a day. If you teach a marketing team the reasons why people churn, they will stop the problem at the source and prevent many lost customers for a lifetime.
Lack of quality data
Artificial intelligence models are like classic cars that require high-octane fuel to run properly. The accuracy of the predictions these models generate depends on that of the data they ingest, which is why the only gas you should ever pump into your AI model is first-party data collected directly from owned digital touchpoints. Unfortunately, not every company has adopted the right data infrastructure tools to consistently collect customer events across every channel that users interact with.
No unified view of the customer
In order to generate recommendations based on a complete view of your users, AI systems require unified customer profiles, not disparate and unresolved events collected from various channels. Many off-the-rack AI solutions skip this requirement, only track activity occurring on a particular device, and calculate insights for that channel only. While convenient, this method doesn't reflect a customer's true history of interaction with your brand across your website, mobile apps, stores and support channels, and therefore can lead to incomplete insights.
Why adopt an AI CDP?
A CDP lays down a foundation of data infrastructure consisting of three key capabilities––collecting high-quality, first-party data across channels, unifying this data into a 360-view of the customer, and forwarding these profiles to downstream tools for activation. This infrastructure is critical to have in place before you can effectively leverage AI insights. By providing these capabilities, an infrastructure CDP becomes the nexus of your entire marketing stack, and generating intelligent attributes with an AI CDP enables you to infuse your entire data ecosystem with this enrichment layer.
An infrastructure CDP is like the Times Square of your data stack (without the slow-moving hoards of tourists). From a transportation standpoint (and literally no other reasons) being in Times Square has its advantages. On the New York City subway system, it’s often easier to get from Times Square to a location in an outer borough than it is to travel between two places in that same borough, even if those places are geographically closer to each other than they are to Times Square. Similarly, intelligent attributes that live on customer profiles in your CDP can seamlessly move to tools for engagement, analytics, attribution, advertising, and back again in bi-directional data flows. Your predictive insights and recommendations become much more versatile and powerful resources than if they were trapped on the periphery of your marketing stack.
Additionally, when you use an AI CDP to generate predictive insights based on unified customer data, you end up with predictions based on a complete picture of your customers. Insights generated within a single downstream data activation tool, however, will be based only on the filtered event and behavioral data that this single tool has access to. This stifles the potential of the insights and limits your growth teams to using them in a single channel of activation, placing omni-channel AI-powered personalization out of reach.
With an AI CDP, you can derive more value from your customer data, and maximize your ROI on data-driven initiatives across your organization. In the next installment of this series, we walk through an end-to-end use case showing how an eCommerce brand can do exactly this leveraging mParticle and Cortex.